Main di J99SLOT bikin tenang karena link-nya resmi dan sudah terdaftar di PAGCOR. Slot Zeus di sini juga paling sering kasih cuan.
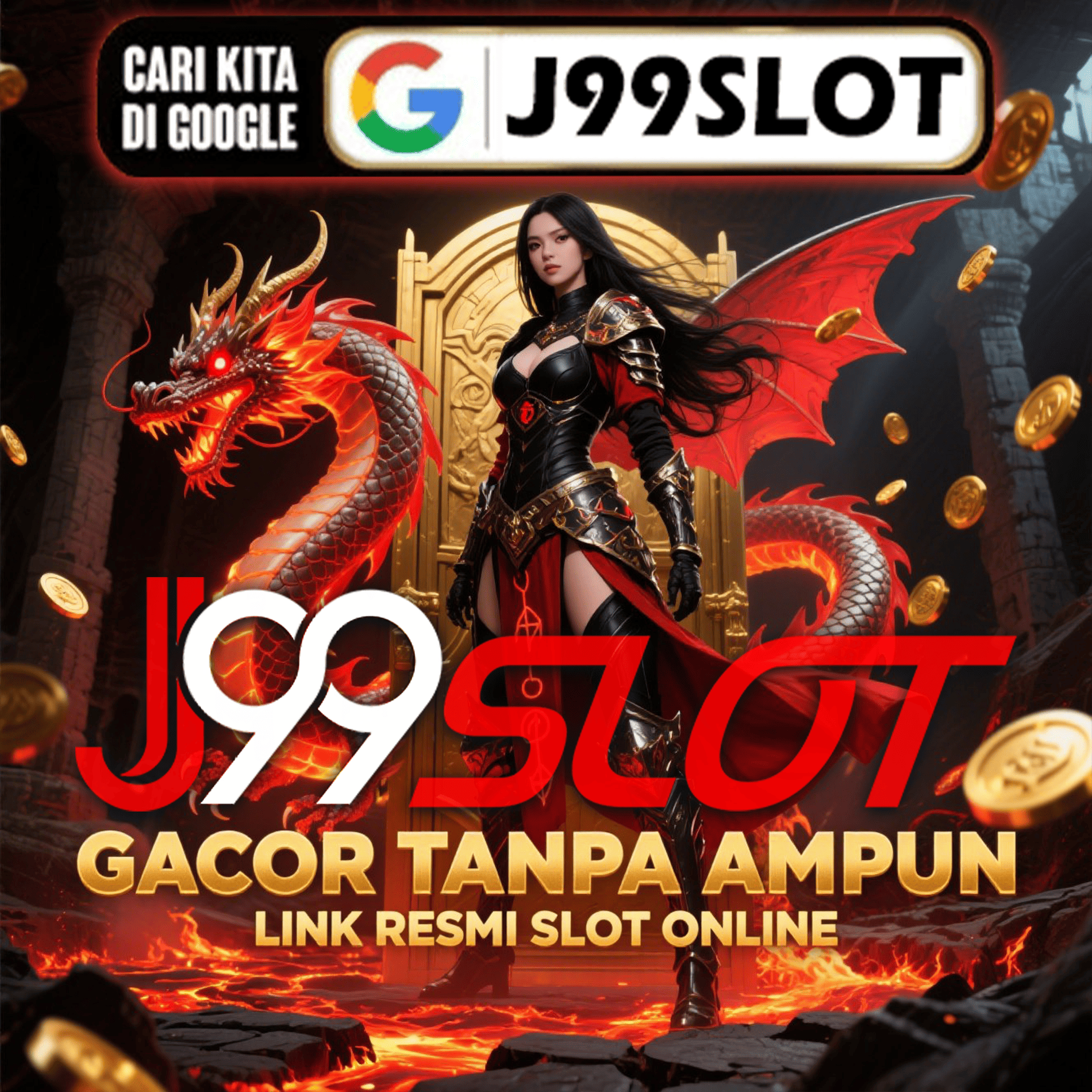
J99SLOT $ Slot Zeus Gacor Online 2025 dari Link Resmi PAGCOR
Podcast
– Original recording
Customer reviews
-
5 star4 star3 star2 star1 star5 star100%100%100%100%100%100%
-
5 star4 star3 star2 star1 star4 star0%0%0%0%0%0%
-
5 star4 star3 star2 star1 star3 star0%0%0%0%0%0%
-
5 star4 star3 star2 star1 star2 star0%0%0%0%0%0%
-
5 star4 star3 star2 star1 star1 star0%0%0%0%0%0%
How are
ratings calculated?
To calculate the
overall star rating and percentage
breakdown by star, we don’t use a simple
average. Instead, our system considers
things like how recent a review is and
if the reviewer bought the item on
Amazon. It also analyzes reviews to
verify trustworthiness.
Reviewed on 21 Juni 2025
Report
Helpful
Reviewed on 20 Juni 2025
J99SLOT memberikan pengalaman bermain yang menyenangkan dan aman. Terdaftar di PAGCOR, jadi tidak perlu ragu. Slot Zeus-nya gacor terus.
Reviewed on 19 Juni 2025